I used to laugh at those TV series and movies where crime investigators could take a surveillance camera photo, however fuzzy and blurred, and enhance its resolution beyond belief to the point where every possible (and impossible) detail becomes visible. I stopped laughing last week at a conference when I became aware of a 2009 study by Moti Segev and colleagues from Technion in Israel that demonstrates an amazing precision in the recovery of objects from imperfect images. The resolution they achieve is better than what even the best photographic lens can ever hope to image.
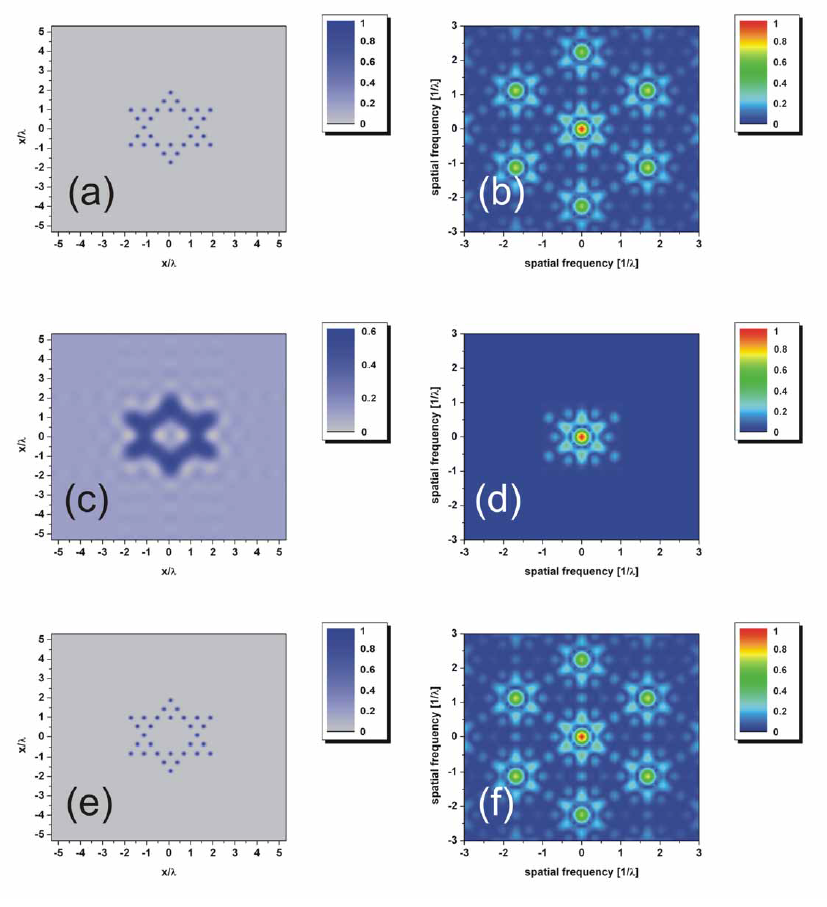
Enhancing image resolution. The top row shows the original object, both the image and its corresponding map showing the different frequencies that make up the image. Middle: the image as it would look like with the best resolution available with optical systems. Bottom: the reconstructed image. (c) Optics Express 17, 23920 (2009)
The notion of such an enhancement seemed so unbelievable at first because there is a maximum in resolution that a classical optical imaging system can deliver. This limit is about half of the wavelength of the light beams used. Any features smaller than this limit simply will not resolve however good the camera lens. Yet Segev and colleagues are easily able to beat this limit – without using any special equipment.
The reason for this limitation is that some of the light – the part that carries the information about the smallest features of an object – is absorbed by the medium through which light travels. Eventually, key information is missing from the image, and no exact mathematical reconstruction is possible.
How do you then reconstruct an image with high resolution? One option is to reconstruct the original object by making assumptions about the information that is missing. For example, if you know that a blurry image consists of the letters of the alphabet it becomes easier to guess the original. But such solutions are always restricted to certain circumstances and are also quite susceptible to noise in images.
Moti Segev and colleagues use a different approach. They have only a single requirement: the assumption that the images are sparse. This means that the image should not contain much information. This is not such a drastic restriction as most images don’t contain that much information anyway, which is why image compression algorithms such as jpeg are so effective.
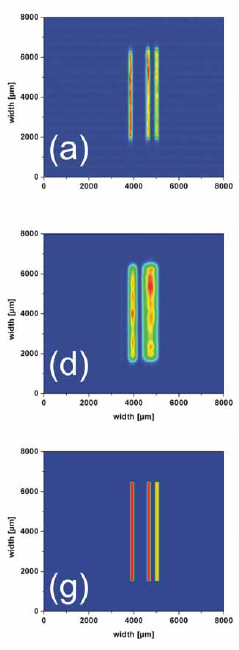
The reconstruction of three lines with different intensity. Top: original image. Middle: The image as seen with conventional optics. Bottom: the reconstructed image even has the right intensities. (c) Optics Express 17, 23920 (2009)
The new mathematical tool Segev and colleagues have developed is partly based on the so-called ‘compressed sensing‘. The algorithm recognises that if you take an image that doesn’t contain much information and add anything further to it, it will become less sparse. Add noise to a white area and it increases the information content of the image. So to reconstruct the original object in the framework of compressed sensing the researchers look for a unique mathematical solution that is consistent with the available information measured and that leads to an image that is as sparse as possible.
This leads to amazing results. For example the Star of David shown above. The original object on the top right gets converted into a mathematically alternative version that shows the different wave components in a two-dimensional map (top right, the so-called Fourier transform). This conversion makes it better to see the impact of the physical limit of an optical lens (middle row): the fuzzy image in the middle row is how a photographic lens would at best be able to resolve an image. In the alternative wave representation, it is obvious that a number of waves are removed, because as discussed these don’t reach the detector (middle right picture). To reconstruct the original object from this reduced image, the computer algorithm now looks for the most sparse solution that is also compatible with the remaining information. And voilà, without any prior knowledge the reconstructed image is an almost perfect copy of the object (bottom row).
The Star of David has some sort of symmetry to it, and one might argue that this helps with the reconstruction. But no, this algorithm works for other scenarios as well, as the example with the three asymmetric lines shows. The reconstructed image even correctly retrieves the relative intensities of the lines! And what’s more, optimising a visible image isn’t where this algorithm stops. What works for light also is good for sound, and this method could also enhance acoustic signals.
Already in this first study on the topic Segev and colleagues achieve image resolutions that by far exceed the fundamental limits of classical optics. The tradeoff of the method is that the maximum resolution that can be achieved is related to how sparse the image is. The less information in the image, the more precise it can be reconstructed. So while this method probably won’t be able to enhance your family photos much, it looks really promising for the typically sparse scientific and technical images. And villain’s number plates of course…
Reference:
Gazit, S., Szameit, A., Eldar, Y., & Segev, M. (2009). Super-resolution and reconstruction of sparse sub-wavelength images Optics Express, 17 (26) DOI: 10.1364/OE.17.023920
This post was chosen as an Editor’s Selection for ResearchBlogging.org
January 15, 2011 at 10:03
Wow, this is amazing, if it pans out. I note though that both the examples they give are artificial test images; do they show that the method works on any kind of real-world images?
January 15, 2011 at 10:50
There is no doubt this pans out! In the talk I heard in the conference last week, Moti Segev and his team demonstrated the recovery of images containing 100nm features: features that are more than 5 times smaller than the optical wavelength, and 3.5 times smaller than the diffraction limit of optics, with the reconstruction accuracy being ~30nm. It is just amazing.
January 18, 2011 at 03:29
So, you’d really just want to take a crappy microscopy image and then turn this algorithm loose? Did Segev et al do much comparison with time/effort/result of using other microscopy techniques (STED, PALM, STORM) and image analyses?
Pretty cool stuff!
January 18, 2011 at 15:02
Yes! And in fact Segev in his talk has shown some preliminary images of this. I’m not saying it is perfect, there are some drawbacks such as the requirement on sparsity. But Segev also said they are collaborating with a big microscope company now. Problem will be doing this in real-time…
January 27, 2011 at 06:57
It is great to see the result of this multidisciplinary collaboration between scientists. Knowing the many facets of both Prof. Eldar and Prof. Segev, and T3 – the dynamic technology transfer unit at Technion – Israel Institute of Technology, one can foresee applications in nanomedicine, research across the frontiers and on. This is a tool that seems to unlock a whole new dimension. Great post. Exciting development!